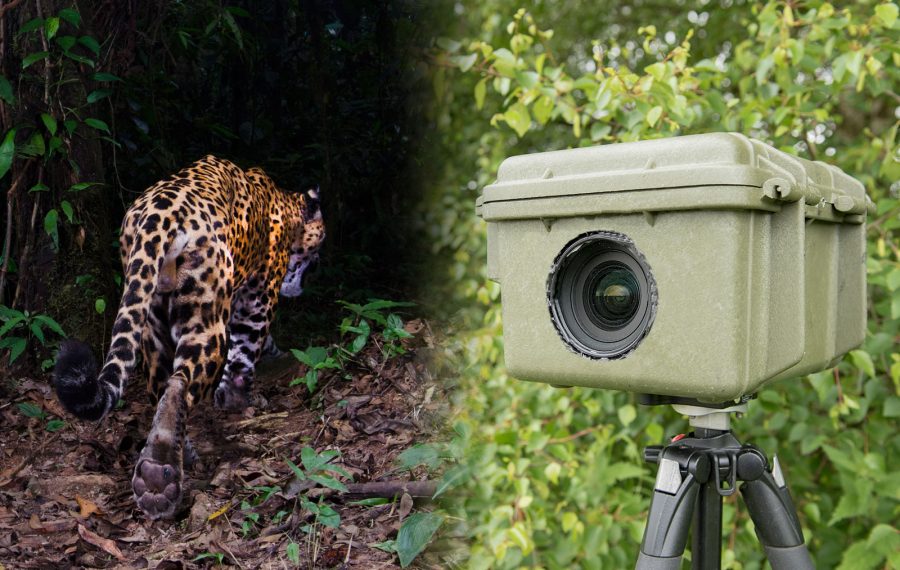
[Summary]
This is an excellent paper demonstrating an end-to-end machine learning pipeline of different modeling tasks to implement an active learning system. Wildlife researchers want to be able to use ML to identify animals from wildlife camera trap images they’ve collected in mass. Their problem is a lack of labeled data to do so effectively.
The burden of manual review is the main disadvantage of camera trap surveys and limits the use of camera traps for large-scale studies.
An ML group set out to help them by developing an active system that labels a largely unlabeled dataset with sparing use of a domain expert’s input (eg asking for labels on specific images that will best help the classifier learn). They’re able to massively reduce the number of labeled images required to achieve reasonable model performance.
The details of the implementation tie together image classification, object detection, transfer learning and embedding learning:
Pipeline Details:
- They first leverage a pre-trained object detection model to prune out images that don’t contain animals, only keeping those that have a +90% confidence of having an animal in the frame.
- The wildlife researchers are interested in the count of animals in the image, so this number is provided, but otherwise not used in the rest of the pipeline.
- Images are cropped to only contain an animal subject and resized to a constant dimension; this reduces the background noise that a classifier might latch onto/overtrain with.
- An “oracle”, or domain expert, labels 1,000 randomly selected cropped images as a seed dataset. These are then embedded to a lower dimensional feature vector with a ResNet-50. Interestingly, they use the “Triplet Loss”, instead of cross-entropy loss, because it encourages better separation in the embedding space between labels. This loss function was popularized in the FaceNet model for creating facial-embeddings.
- Lastly, a simple 2-layer DNN acts as the species classifier on the feature vector.
Active Learning Loop:
- Select 100 images that, according to a criteria, will provide the most information to the model if they had labels, and sends them to an oracle for labeling. These are then embedded and the classifier is retrained. This constitutes a “step”.
- The criteria for selecting what data to have labeled the oracle is key to the active learning system. They evaluate 2 approaches: A model-based approach of choosing samples yielding the highest model uncertainty, or a data-based approach that samples data in a way that it is representative of the underlying distribution.
- After every 20 of these above steps, and also attaining 2,000 labeled images, retrain the embedding model. They note that model performance jumps each time this is performed.
- This is repeated until a satisfactory performance is achieved on a test set. They now have a model that can confidently classify animals from this set of camera traps.
Results:
- They achieve 91% accuracy with 95% less data (labeled images). This is probably due in part to the process described above, but also the selection criteria of images that are labeled by the oracle.
[Commentary]
Many ML applications are stifled by a lack of labeled data, or a high cost to collecting those labels, so active learning approaches like this will expand the space of possibilities. A 95% reduction in data labeling costs would yield more time for tweaking modeling techniques, or potentially create much larger and diverse datasets. Because the active-learning process is selecting the “best” images for human labeling, the quality of the seed dataset is higher. Importantly, it also now requires a less complex model architecture to achieve a reasonable performance threshold, allowing engineers more time to fine-tune their model.
#GlobalAIandDataScience#GlobalDataScience