In this post, I will discuss the changes that organisations need to implement to truly benefit from data and AI. Part 1 of this series, focused on setting up the stage and discussing the aspects that make a business data-driven. While in Part 2, I have discussed the challenges that keep the businesses away from becoming data-driven.
What are the main challenges for companies to solve in their pursuit to become truly data-driven?
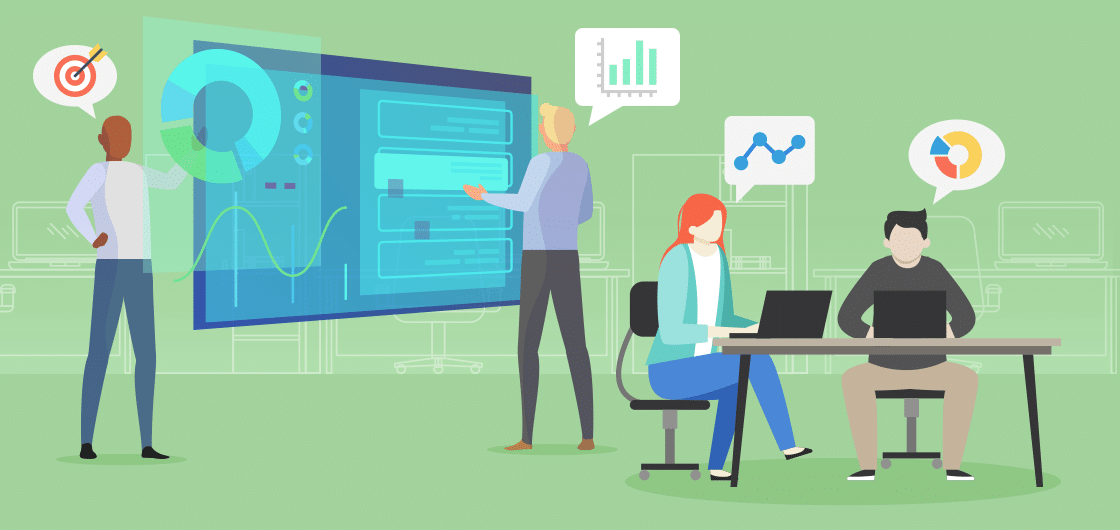
There are many changes that businesses need to implement, and they vary from business to business. I can spend hours on this, but let me give you some gist of this.
- Look at the bigger picture: Stop looking at only the points that you can see right now, try to look beyond those points and think about what will come next. Use your imagination, look at your competition and try to look at the big picture that entails a future based on intelligent automation, artificial intelligence and their likes driving and thriving the businesses. Then, decide are you willing to be out-thrown from the market, or would you like to stay relevant for the time to come. In the later case, which most, if not all would like to do, take the necessary steps to ensure that you develop plans, set right strategic priorities and take actions to lead your organization to land as a cognitive enterprise.
- Develop Shared Ownership and Share Vision across the organisation: Carrying further from the above point, once you have started to take steps in that direction, start sharing your vision across the organisation and do it repeatedly, so that everyone within your organisation owns that vision for the future. Once, they own it, it will become much easier to get them to do things in accordance with that vision. However, this many not be as easy as it sounds, you might have to look deeper and find out what kind of cultural changes, do I need to implement across my organization. Once you have found the needed changes, don’t hesitate from implementing them even if they may offer some risks and financial implications.
- Move from Return on Investment (ROI) to Return of Value (RoV): Take necessary measures to ensure that you and your organisation moves away from ROI mindset to a RoV approach. This will ensure that when your Finance department and Chief Financial Officers (CFOs) are coming in the way of releasing the funds for your business units to implement data science and AI, you hold their hands and show them the brighter end of data and AI and let them realize and think about RoV of such spendings on the data and AI endeavours.
- Address the Lack of skills gap:
Make plans to fill the skills gap, some suggestions would be
- Hire contractors to fill the short term need: As it is not easy to hire skilled data scientists at short notice (especially when you need them), the short and quick solution would be to hire specialists (contractors) to achieve short term objectives of the proof of concept (POCs) to get buy in across your organization.
- UpSkill or Re-skill your workforce to adapt to and learn the necessary skills: Look out for the enthusiasts within your organization as well as people who needs to be reskilled (such as data owners, business unit leaders and then everyone in the organization in in this descending order of priority). Get them all trained as per their responsibilities and potential involvement in the data and AI efforts. Best food is the one which is cooked at home, keep training your people in house.
- Partner with third parties and do projects in a way that ensures that your team is also trained on the go: Engage with consulting organisations and third parties on defined, concrete and measurable projects. Ensure that, those consultants also engage your employees for the knowledge handover, sharing of work and co-creating the data science POCs.
- Data access and preparation:
Data access is limited usually by two factors:
- Data Literacy which we have discussed earlier in Part 2 of this series,
- Data owners not giving access
There are additional measures that you can take to address the issues faced in data access and data preparation
- Data Dictionary: Build a data dictionary or Knowledge Catalog, to ensure that everybody is on the same page across the whole organization. This agreement on what does a specific term mean and what does it stand for would help you avoid many problems down the road. One of the main issues that it will address is the knowledge gap, should some of your employees leave, retire or transfer out of their present role.
- Joint workshops for the employees of different business units to let them feel the need and value that they could get from data and AI projects: Put people from different unity together to brainstorm the ideas, and discuss the potential opportunities for data and AI initiates (projects). This will help them feel being involved, learn from each other and take ownership for data and AI projects.
- Step away from budget centric to Value Creation mindset: Thought that you have limited budget:Look for opportunities, for optimising existing processes, resource utilisation, cutting down the costs, and creating new avenues for your business. Once you change this thinking, you will aim for ways to look for opportunities to create or arrange budget for data science initiatives instead of sticking to “we don’t have money” approach. You and your employees will be then ready to go extra mile to support, and evangelise data and AI efforts.
- Architecture or Infrastructure Landscape: What architectural or infrastructural changes are needed to get the full benefit of AI and Data. It might happen that you do not need (in most cases) any changes on those fronts as you are starting with Proof of Concepts/ Values in the beginning but it would be helpful to start preparing early on. As you and your team becomes mature and expert in utilising the promise and potential of data and AI, the next steps that you would like to take are to deploy the Machine Learning models in production environments which can handle scalability and flexibility for the business and its users.
In the next blog, I will discuss about what implications would surface for the business, once they start their journey to become a data driven enterprise.
#GlobalAIandDataScience#GlobalDataScience#Highlights-home