The benefits of accurate forecasting are widely understood and can play an important part for organisations to fulfil customer demands, optimizing inventory and achieving revenue targets.
There are many forecasting approaches available and influenced by factors such as the purpose of the forecast, data available and level of sophistication required.
Let’s take a manufacturer as an example. They may have some clean, high quality, historical data that is representative for the future periods that a forecast is required for. This situation may be best suited to a time series approach where seasonality and trend are evaluated to extrapolate into the future.
By contrast, identifying and collecting appropriate data may require more work such as data mining. For example, joining data from disparate sources, dealing with missing values and outliers and so on. The manufacturer may also require multi-variant factors within the forecast calculations such as new product mixes, price and cost causality, weather predictions.
IBM Planning Analytics enables business users to automatically generate time series forecasts including a range of easy-to-understand options to influence the results. This approach can also be augmented with a data science project to address the data challenges and forecast techniques described above.
Here’s an example using IBM Planning Analytics.
GET DATA
Data can be imported from source systems and/or gathered directly using the solution’s contribution & workflow capability. If there are no issues (e.g. missing data, exceptionally high/low values, etc.) the user could go directly to generating the forecast. However, in this example the user would like to correct some anomalies.
- The initial data looks representative for the time horizon forecast apart from two periods (Pd7 and Pd17) based on business knowledge
- For Pd7, Avg is selected which automatically calculates an average value based on Pd6 and Pd8
- For Pd17, the original value was considered an outlier and is overridden with the value of 130
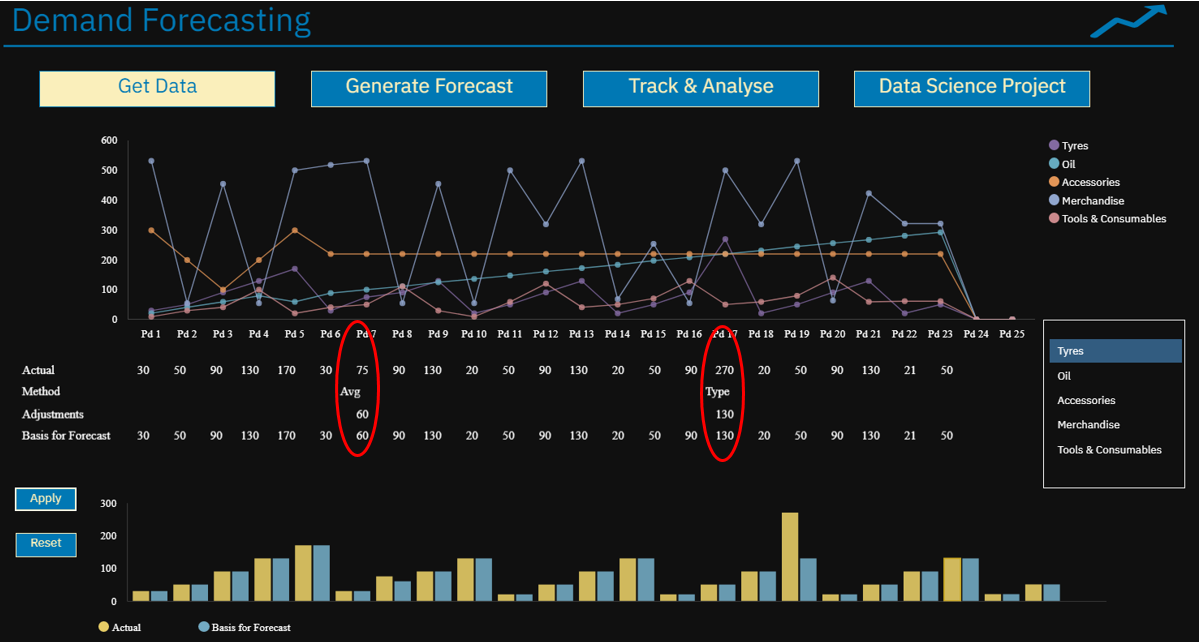
GENERATE FORECAST
The business user selects the product (s) and the time series forecast is automatically generated using the highest scoring model.
- The user was able to select the range of data used, whether seasonality should be detected, confidence intervals and how many periods to forecast. This instant forecast is user. data and timestamped including the chosen parameters.
- Actuals for Pd24 are subsequently loaded, forecast accuracy is automatically calculated and the user elects to override the forecast for the next period.
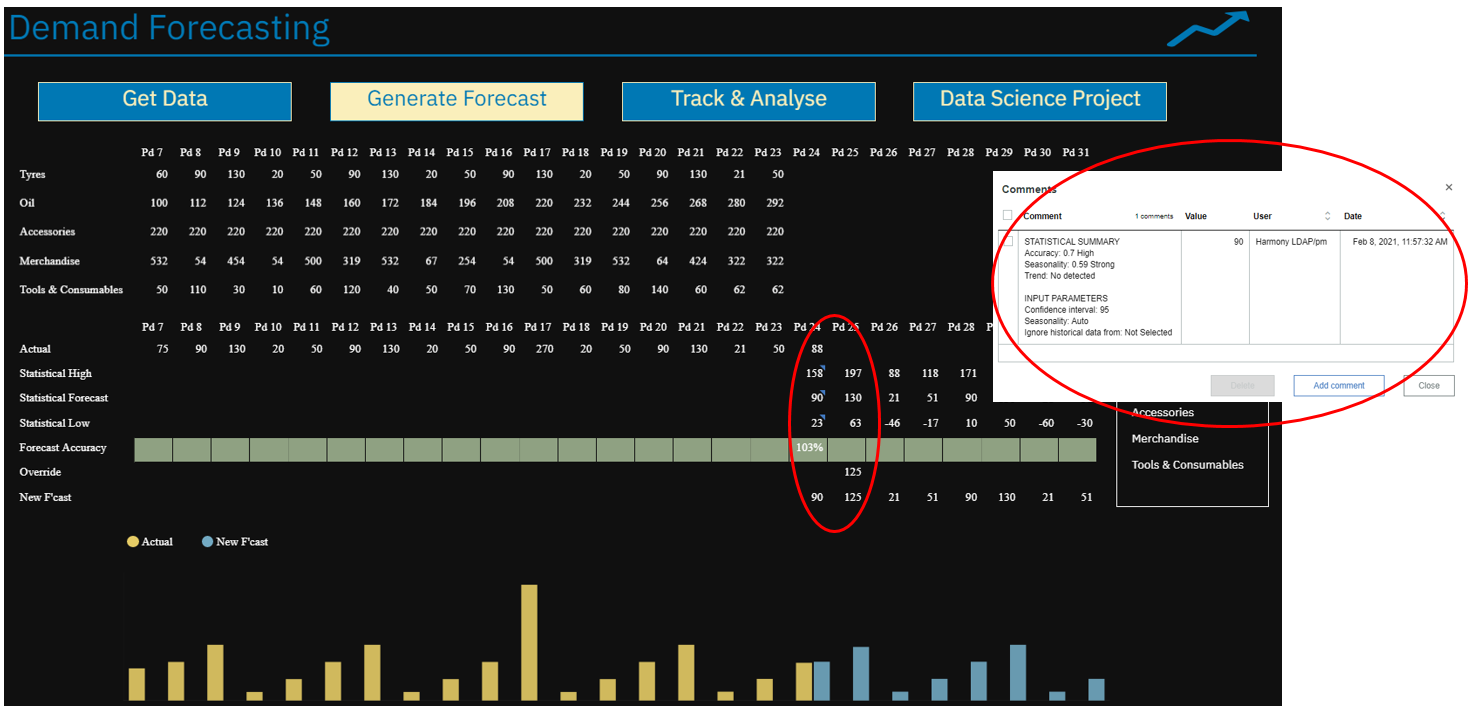
TRACK & ANALYSE
As well as monitoring the forecast accuracy other versions of the plan are tracked accordingly. Some of these plans are facilitated directly within IBM Planning Analytics, e.g. Budget, Plan, Actuals, Forecast and so on. Other plans are imported from data science projects, e.g. Python (open source) and Watson Studio.
This time series forecasting example enables the business user to select parameters, enter adjustments (pre and post forecast), track accuracy, compare against other generated plans and take further action.
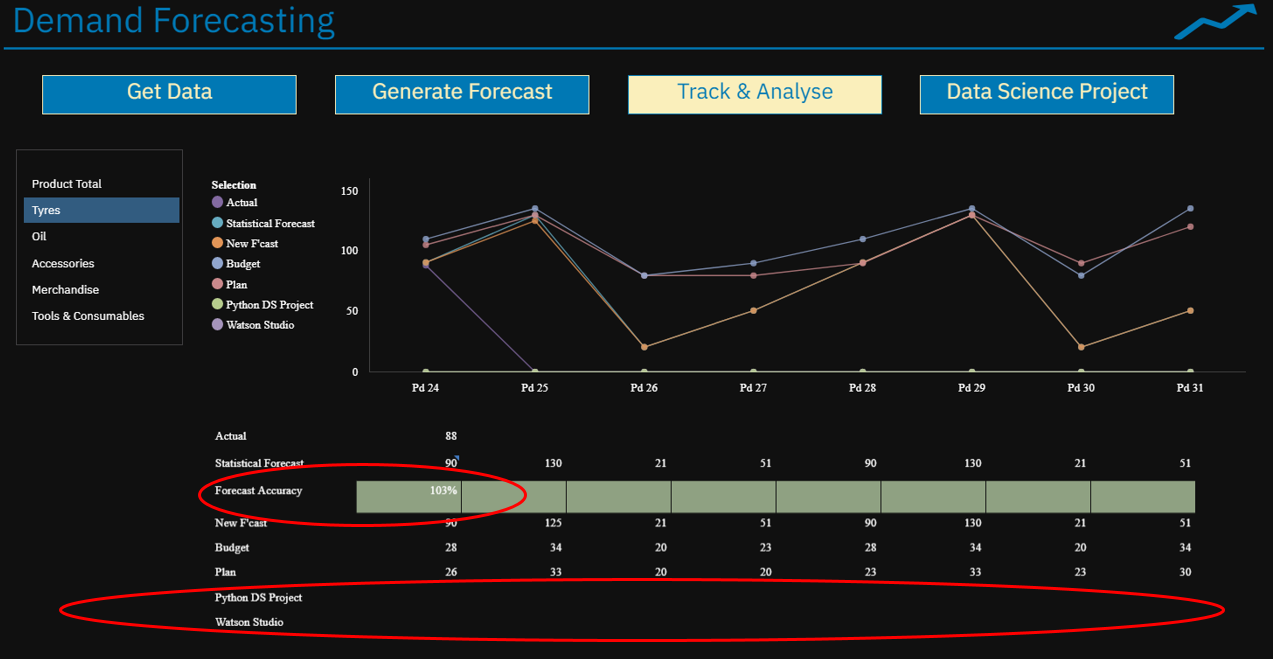
Here are the main considerations for time series forecasting using IBM Planning Analytics.
- Collect/load historical data
- Ensure historical data is over a continuous and sequential time horizon
- Adjust/override any past time periods if required
- Select range of data to be used as a basis for statistical forecast
- Results can be more effective if historical data spans at least double the forecast time, e.g. 3 years of actuals to produce an 18-month forecast
- Enable/disable seasonality detection as required
- Select confidence interval to determine upper and lower boundaries of forecast projection
- Resulting forecast projection user/date/timestamps summary of statistical forecast approach
- Compare forecast with other versions of the plan, e.g. top-down, bottom up, imports from data science tools such as Watson Studio or Python, etc.
- Adjust/override any future time periods as required
- Track forecast accuracy retrospectively and going forwards
- Increase re-forecast frequency
Please see here for more detail on ai-infused forecasting using IBM Planning Analytics.
DATA SCIENCE PROJECT
If you have data challenges or data science project requirements, then IBM Planning Analytics can integrate with those projects. It is also available with IBM Cloud Pak® for Data which is a fully integrated data and AI platform.
===============================================
#PlanningAnalyticswithWatson