Now that data is recognized as the new currency of the 21st-century, businesses are doing everything they can to acquire it. They collect it from customers. They mine it from their social media accounts. They'll even purchase it from data brokers and aggregators. They've become experts at pulling data from every aspect of their operations.
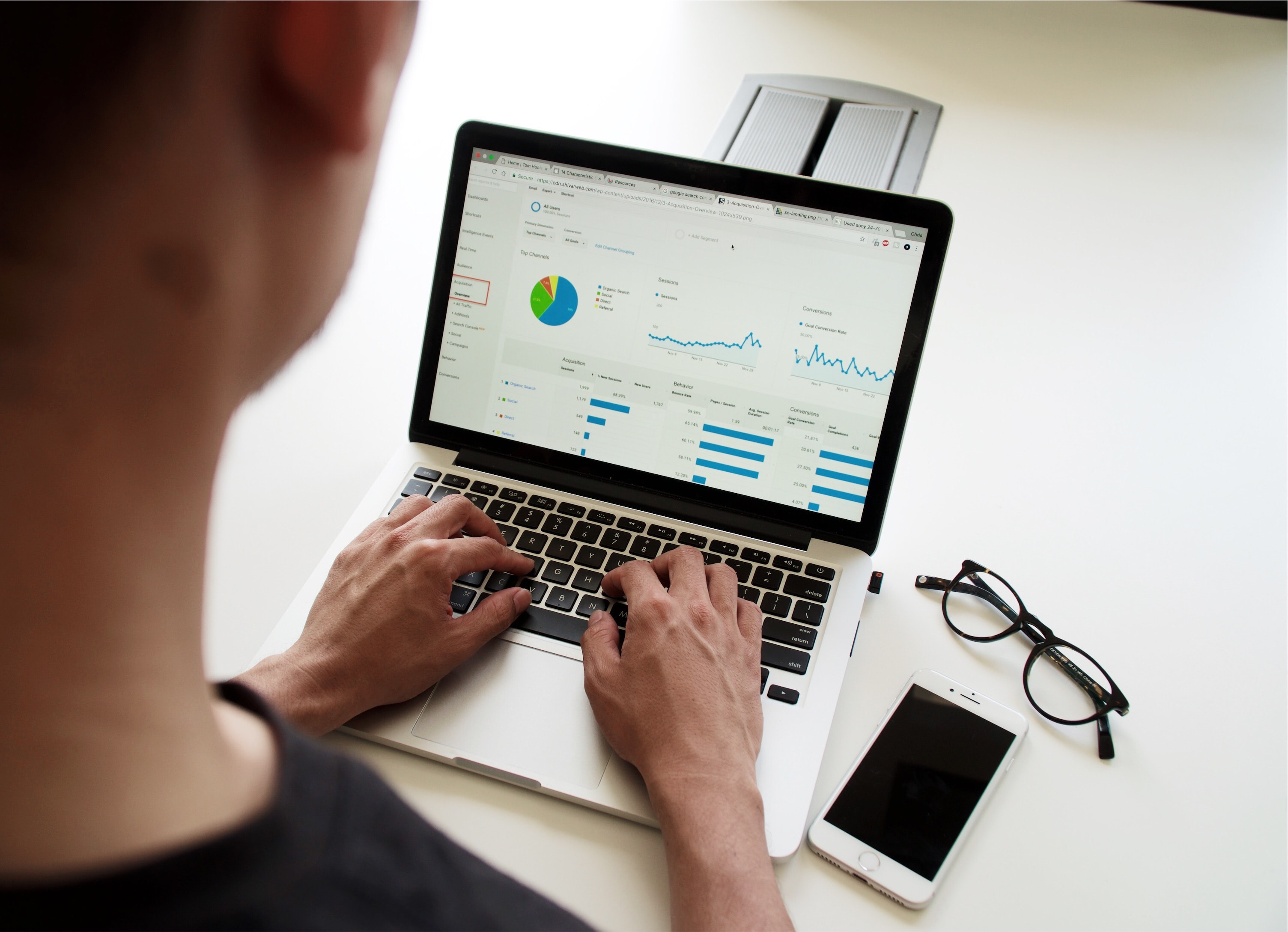
But having such varied data coming from so many sources creates a major challenge. It's that making sure that all of the data is of sufficient quality to be useful is getting harder by the day. As it is, data scientists now spend up to a quarter of their time cleaning data to prep it for use. And believe it or not – that's an improvement.
It used to consume up to 80% of the average data scientist's day in the recent past. But better data cleaning tools like IBM Watson's Data Refinery have chipped away at the most time-consuming parts of the process. Even so, there's still a long way to go.
And that's where automation enters the picture. With each passing day, more AI and ML tools become available to data teams to help them automate more of their data cleansing processes. And while they're still imperfect solutions, they can help data teams free up more of their time to do the work necessary to turn data into meaningful insights. Here are three of the places that AI and ML can be useful in automating data cleansing as of now.
Outlier Identification
One of the most necessary parts of any data cleansing operation is a workflow to identify outliers in the data. This then makes it possible to categorize those outliers to decide if they represent meaningful variances or are simple data entry errors or other mistakes. And a properly-trained ML model can make the automation of the whole task a reality.
This is accomplished by training the model with representative sets of data that have already been cleaned according to the data team's specifications. The ML model will then be able to replicate their outlier selection process with future data sets and apply the needed mitigation techniques as necessary.
Deduplication via Clustering
Another part of the data cleansing process that makes an excellent candidate for ML or AI-driven automation is the deduplication process. This is one of the more time-consuming tasks that data teams contend with, and one that isn't easily automated through simple scripting. This is because scripted approaches often miss duplicates when they contain omissions or errors and don't represent exact matches.
For example, a business combining an internal customer email list with one purchased from a vendor like Bookyourdata might have a significant overlap that would be missed by a script-only approach. This is especially the case if the purchased or internal data wasn't subject to pre-validation (through server response tests and the like). In such situations, a simple mistyped character could result in having two versions of the same email address make it into the final data set. But an ML model can look for those instances and reliably identify them – resulting in a smaller cluster for the data team to check.
Merge/Purge Scoring
After the deduplication process is complete, it can still be quite a bit of work for data teams to decide on what to do with the resulting clustered data. But ML models can also help to automate that part of the process somewhat by creating reliable scores to guide their decision-making. By tagging each bit of data with a confidence score that indicates the likelihood that it belongs in the cluster, the model can speed along the merge/purge workflow that's needed to resolve any remaining inconsistencies.
In some cases, it may even be possible to fine-tune the ML scoring model to the point where it can handle the merge/purge process without intervention. This is often the case when dealing with data sets that get updated or amended over time. When the data sources remain the same, it becomes easier to train an ML to make high-confidence decisions based on historical performance.
No Complete Shortcuts
It's worth pointing out that it's still impossible to automate every part of a data cleansing operation. There will always be wildcards involved that today's ML solutions can't cope with, and data teams do still have to be careful how they're applying ML and AI-driven automation. But that doesn't mean it isn't worth trying to automate as much as is feasible.
And the rewards involved are huge. With data scientists still spending so much of their valuable time on data preparation tasks like cleansing, even minor time-savers are a major help. And, as ML solutions continue to improve, they're only going to become more capable of shouldering the data cleansing burden. By starting to automate parts of the process now, businesses end up with workflows that are easier to turn over to complete automation in the long run. And that alone makes data cleaning automation worth exploring right away.
#DataSet#MachineLearning#MasterDataManagement#data_manipulation