FakerFact: Fake News Detection for the Modern Age
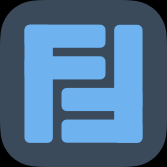
For the past 3 years, I’ve co-engineered a project called FakerFact. FakerFact is a tool to help users discern journalistic and credible news content in the huge selection of media we consume in this age of “fake news.” The tool is not developed to tell readers what they should and should not be reading, or even to censor certain content; rather, our goal is to empower readers to make their own choice of what to read, by checking a set of scores given to content based on a number of analyzed dimensions about that content.
The core of the project is natural language processing experimentation, employing state-of-the-art deep learning techniques. By appropriately aligning the signals we look for in natural language, like writing style, we’re able to redefine what seems like an intractable problem (automated truth detection) into one we can make an impact on, namely, detecting when an article is trying to manipulate the reader with more than just the facts. The story of our journey through fake news, our underlying motivations why we built the project, and the use cases are outlined in this first part. We’ll follow-up with the data collection, modeling, and deployment in another series post.
Our Hypothesis About Misleading Content:
“Fake News” is a recently coined term, referring to content online intended to sway our beliefs about a topic with certain claims. It’s problematic because not only does it propagate quickly among people, it’s also hard to verify claims made within articles. In fact, fake news is 70% more likely to get shared on Twitter than true stories, and it takes true stories 6x longer to propagate to a sample population. Gauging the truthfulness or factuality of an article is difficult because news is new, and the availability of the ground-truth may be hard to pin down. It’s unlikely that we can generate an AI to determine what is true given this challenge, and our goal is not to make a truth detection engine.
Looking to realign fake news detection then, we might want to identify when there are hidden intentions behind an article, or intentions trying to manipulate or sway our thinking in a certain direction. A less trustworthy source of information attempts to hijack a readers’ emotions in order to overrule rational thinking and cause the reader to overlook stated claims.
Therein lies our hypothesis:
There exists underlying signals in less reputable content that try to elicit an emotional response from us. We believe that sophisticated modeling techniques can detect these signals, and be used as proxy signals for the trustworthiness of content.
Looking at psychology case studies [1] [2], we know that when someone is emotionally engaged, their cognitive functioning declines. This is because humans have evolved to not overthink in certain circumstances when emotions are activated as a basic survival instinct.
Effectively, malicious fake news takes advantage of this.
But this activity is something we demonstrate is detectable with deep learning. Furthermore, unlike fact-checking, this type of analysis is scalable. It reduces the risk of claiming something is unfactual, when it is indeed true or half-true. Good journalism is tasked with conveying credible information, and frequently does so without emotional manipulation. Malicious fake news attempts to pedal false claims with an envelope of emotional manipulation.
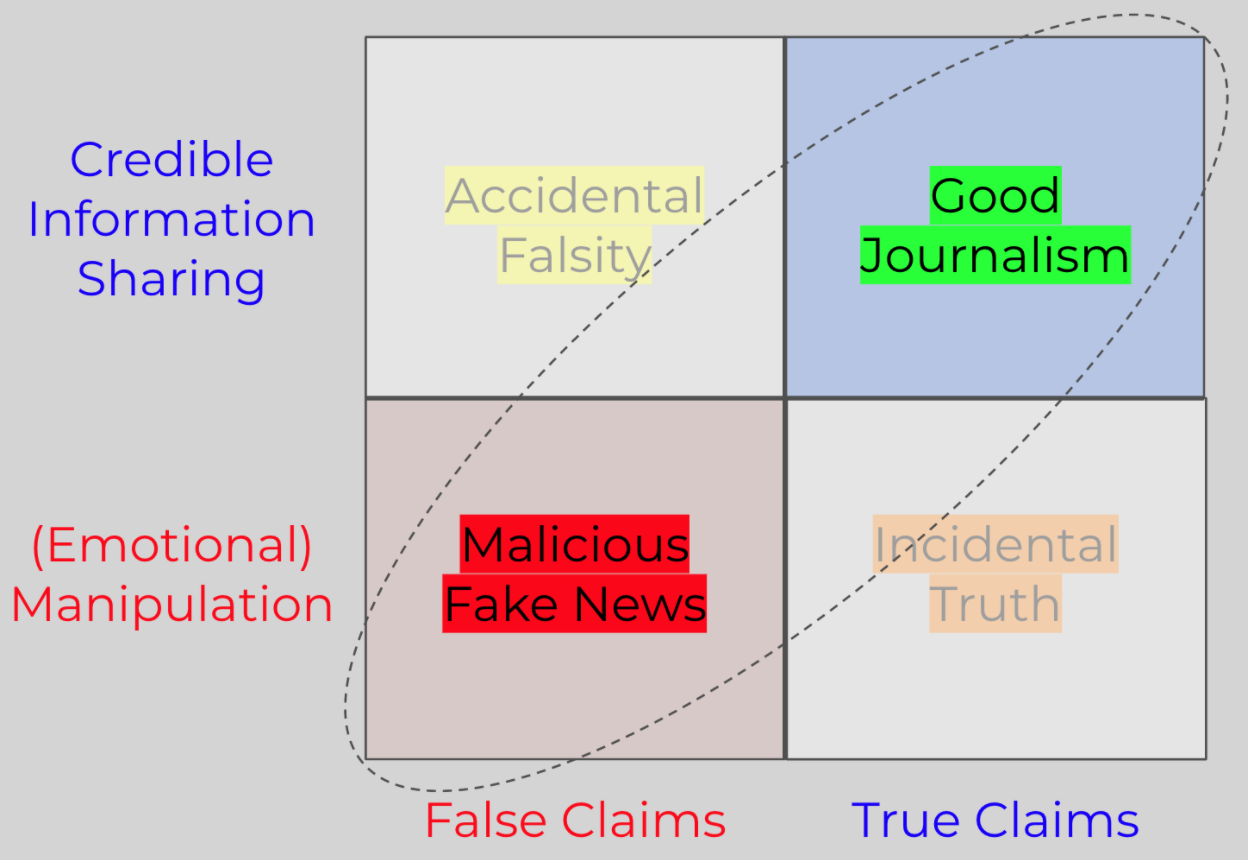
Motivation:
FakerFact’s goal is not to tell online users what they should and should not be reading. We think content consumption habits are akin to consuming food. You are free to consume sugary foods, like ice-cream, but you probably don’t want to consume them excessively. To consume in a healthy and balanced way, you read the nutrition label.
FakerFact is your nutritional label for online content
It can be pleasurable to read sensational content, but it’s also important to know when we’re reading something sensational vs journalistic, or content parading as journalistic but is actually agenda-driven. Continuing the food analogy, an individual item of food is comprised of multiple factors (fat, carbs, protein), and a written piece also contains different styles, literary techniques, and voices. Understanding the proportions of all the factors of trustworthiness present in a single article helps balance individual consumption.
FakerFact aims to be a small part of the fight against fake news by empowering you to make informed decisions. The value of where you choose to guide your eyes is paramount, your influence on your social network is undeniable. Knowledge is power and you can help decide who has power over the news cycle.
The Solution:
FakerFact’s primary offering provides the nutritional content of the writing along the below dimensions. However, for machine learning systems to be reliable and trustable, they must provide interpretable proof for their classification. FakerFact extracts the evidence from an article that our algorithm, that we named “Walt,” paid the most attention to while calculating its answers in order to further help readers come to their own conclusion.
FakerFact’s Walt is most easily accessible as a Chrome and Firefox extension. Alternatively, Walt can also be used without any installation by copy-pasting text into our engine input.
A Taxonomy of Online Content:
We’ve aligned our national fact components along the below dimensions. We believe that they give relatively unbiased and objective assessments of the writing style you’re considering consuming. A scored piece of content has a 0-100 score in each of the dimensions, since like in topic modeling, an individual document is drawn from many different topic distributions.
- Journalism
- Journalism exists to share information. Articles do not attempt to persuade or influence the reader by means other than presentation of facts. Journalistic articles avoid opinionated, sensational or suspect commentary. Good journalism does not draw conclusions for the reader unless manifestly supported by the presented evidence. Journalistic articles can make mistakes, including reporting statements that are later discovered to be false, however the mark of good journalism is responsiveness to new information (via a follow-up article or a retraction), especially if it countermands previously reported claims.
- Wiki
- Like Journalism, the primary purpose of Wiki articles is to inform the reader. Wiki articles do not attempt to persuade or influence readers by means other than the presentation of facts. Wiki articles tend to be pedagogical or encyclopedic in nature, focusing on scientific evidence and known or well-studied content, and will highlight when a claim or an interpretation is controversial or under dispute. Like Journalism, Wiki articles are responsive to new information and will correct or retract prior claims when new evidence is available.
- Satire
- Satirical articles are characteristically humorous, leveraging exaggeration, absurdity, or irony often intending to critique or ridicule a target. Claims in works of satire may be intentionally false or misleading, tacitly presupposing the use of exaggeration or absurdity as a rhetorical technique. Satirical articles can often be written in a journalistic voice or style for humorous intent.
- Example Article
- Walts Opinion
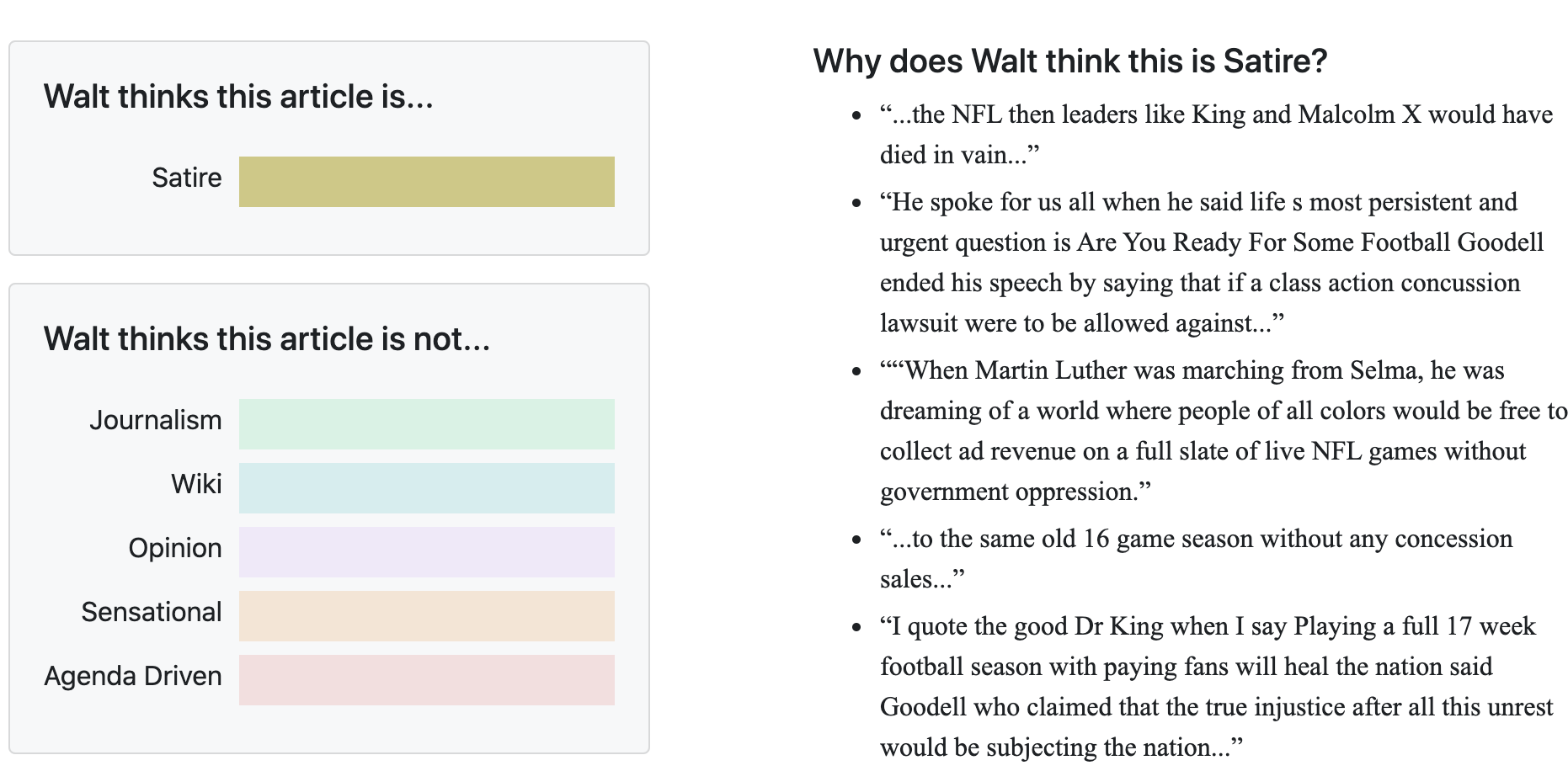
- Sensational
- Sensational articles provoke public interest or excitement in a given subject matter. Sensational articles tend to leverage emotionally charged language, imagery, or characterizations to achieve this goal. While sensational articles do not necessarily make false claims, informing the reader is not the primary goal, and the presentation of claims made in sensational articles can often be at the expense of accuracy.
- Example article
- Walt's Opinion
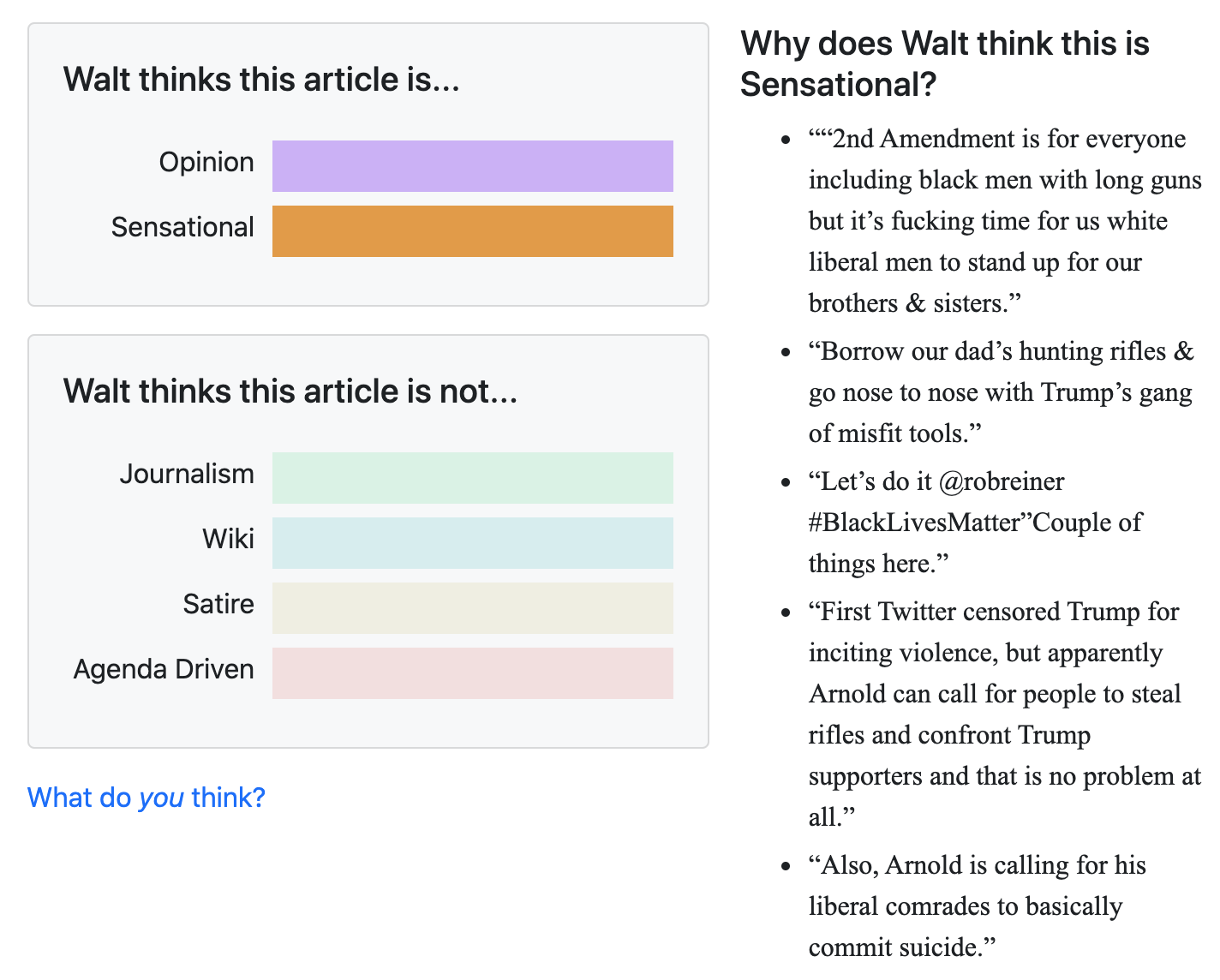
- Opinion
- Opinion pieces represent the author’s judgments about a particular subject matter that are not necessarily based on facts or evidence. Opinion pieces can be written in a journalistic style (as in “Op-Ed” sections of news publications). Claims made in opinion pieces may not be verifiable by evidence or may draw conclusions that are not materially supported by the available facts. Opinion pieces may or may not be political in nature, but often advocate for a particular position on a controversial topic or polarized debate.
- Agenda-driven
- Agenda-driven articles are primarily written with the intent to persuade, influence, or manipulate the reader to adopt certain conclusions. Agenda-driven articles may or may not be malicious in nature, but characteristically do not convince the reader by means of fact-based argumentation or a neutral presentation of evidence. Agenda-driven articles tend to be less reliable or more suspect than fact-based journalism, and an author of agenda-driven material may be less responsive to making corrections or drawing different conclusions when presented with new information.
Use Cases
With this redefined understanding of fake news and our motivation, what is it that we could achieve with an algorithm capable of providing this insight?
Ourselves
First, we can help individuals become better-informed consumers of information. This will help reduce the polarity we see today in political and ideological settings where emotions are distorting people’s ability to draw factual conclusions. In turn, this leads to more thoughtful dialogue, and drives us towards creating better informed, diplomatic solutions to our societal issues.
Sharing with Others
Pragmatically, a tool like this limits the spread of less trustworthy and agenda-driven content. Human nature is to share novel information with one another. It’s reasonable that we also want to avoid sharing unfactual information (unless of course the individual has an underlying motivation). If we provide people with nutritional facts about content they plan to share, they can use that information to reduce the chances they share something unfactual or emotionally manipulated.
Stopping it at the Source
Helping readers is not the only avenue for addressing fake news: perhaps we can help journalists push back against an unfortunate trend we’ve observed that makes the detection of motive driven fake news harder. Journalistic content writers are adopting a performance / story-telling type approach in order to make their information more popular and influential, frequently transgressing the cited emotional rails. It’s unsurprising why this occurs: In a competitive landscape for attention, who doesn’t want their information to be more influential? This tactic is fine for entertainment, but it should not be misconstrued with simple fact-based information conveyance. By allowing journalists to grade their content with this tool, perhaps they will move away from emotionally driven content that purports to be written with journalistic integrity.
Follow the Money
Lastly, building off the assumption that associating and sharing untrustworthy information hurts your reputation, leads us to the final use case, and perhaps most effective. Corporations spend massive amounts of money building, maintaining, and protecting their brand reputation. Companies actively associate their brand with online content that readers consume through advertising, and are concerned about showcasing their brand next to questionable or inflammatory content since it can cause consumers to disengage with their products. This is a great use case for our technology to solve.
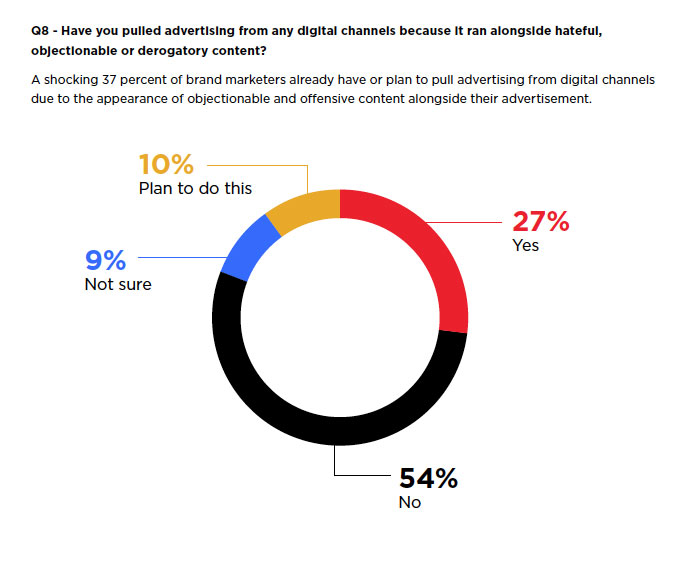
Source: Brand Protection From Digital Content Infection
Online advertising is conducted through nearly all-automated ad-marketplaces, and corporations are not able to vet every page an ad may land on. This leads to a strategy of blacklisting and whitelisting entire domains for advertising. But, as we’ll discuss later in our lessons learned from data collection, labeling a single domain as unacceptable is not useful - domains frequently contain mixed-signal content. By pinpointing content that has an unacceptable nutrition label to a brand, companies can select the level of exposure they’re willing to take and increase the available space for advertising.
Because money moves decisions, this might encourage journalistic writing while discouraging less trustworthy material since it will generate less revenue. Online ad spending topped $333 billion last year. Influencing where this money goes will make a dent in the prevalence of fake news.
We’re constantly on the lookout for more applications of FakerFact that can make the web a better place. If you have some thoughts about use cases we might not have considered, we’d love to hear from you. Our next series post will further discuss how we built this engine, and the datasets we’ve created.
#GlobalAIandDataScience#GlobalDataScience#Highlights#Highlights-home#News-DS