There are many conversations on the topic of bias happening wherever data science and in particular machine learning or deep learning are discussed.
In this blog I want to explore the topic from a different perspective, one that was inspired by Hilary Parker (Data Scientist at Stich Fix) at WiDS@Stanford University.
It was a fascinating and empowering day, these women were deeply technical and at the same time possessed incredible executive gravitas. The topics ranged from “Using Data Effectively: Beyond Art and Science" to the "Evolution of Machine Learning for NIF Optics Inspection" (Watch WiDS 2019 Stanford Conference Videos here).
Listening to the problems they were exposing and the solutions they were proposing, it was hard not to be reminded about the power that data science holds, and therefore the power data scientists have in their hands. They determine, by virtue of the models you choose and or build, the data collection process and your own understanding of the problem, the treatment a patient receives, if a person goes to jail, or who receives credit. And this has made bias a frequent topic in the news.
Hilary talked about looking at the data science process as a system and thinking about its design from the data collection process all the way to communicating results. She talked about the importance of using Design Thinkingprinciples and therefore developing EMPATHY. She stated EMPATHY, contrary to what people might think, can be cultivated. And she urged data scientists to do it.
EMPATHY allows data scientists to put themselves in other people’s shoes and connect with how they might feel about their problem, circumstance or situation. It helps a data scientist set aside his or her own assumptions about the world in order to gain insights into another person or group’s needs. It translates into inherent knowledge of the business, the customers and their needs and aspirations, and all the participants in the data science cycle. It helps them be better at their job.
Bias creeps in at various points of the data science cycle (see figure): when framing the problem, when the data is being collected, when the data is being prepared, when a model is selected to represent the data, or how the results change as the model degrades and results are visualized and communicated.
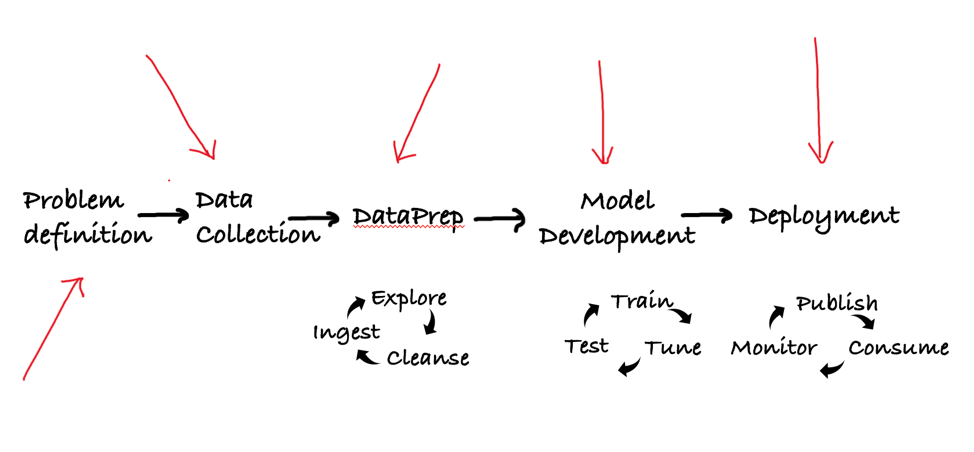
If bias can creep in at any point in the data science workflow, then Hilary’s proposal that you, the data scientist, must look at it as a system — think about the entire process and the people you are interacting with end to end, becomes more compelling. Being thoughtful to attempt to identify potential biases at any point in the data science cycle.
Developing EMPATHY then becomes an imperative to prevent bias: empathy with the subject(s) who will be impacted by the decision a model makes, empathy with the business leaders and their objectives, the data engineer and their challenge and the I/T department.
Hilary sated there are many ways to develop empathy; the most effective for her has been MINDFULNESS and MEDITATION.
Mindfulness helps people excel at their job. It’s been shown to help with decision making, memory and listening skills — with stress reduction and increased productivity.
I know the impact mindfulness has in the workplace and in people’s lives: can mindfulness be a powerful tool in a data scientists toolbox to deal with bias?
As a data scientist you are under a lot of pressure: the expectation of what data science can do for a business, coupled with the speed and increasing number of projects, the concerns over bias….do you struggle with communicating your results? Or with the frustration of the data clean up process? The stress of deadlines and unavailable data?
How do you deal with the pressure today? If you were given data that showed you how mindfulness can help you excel, would you try?
#Article#Bias#datascience#GlobalAIandDataScience#GlobalDataScience