The first open-source foundation model for time series forecasting, Lag-Llama, is released!
Discover here how chatbots progress from predicting words to forecasting the future.
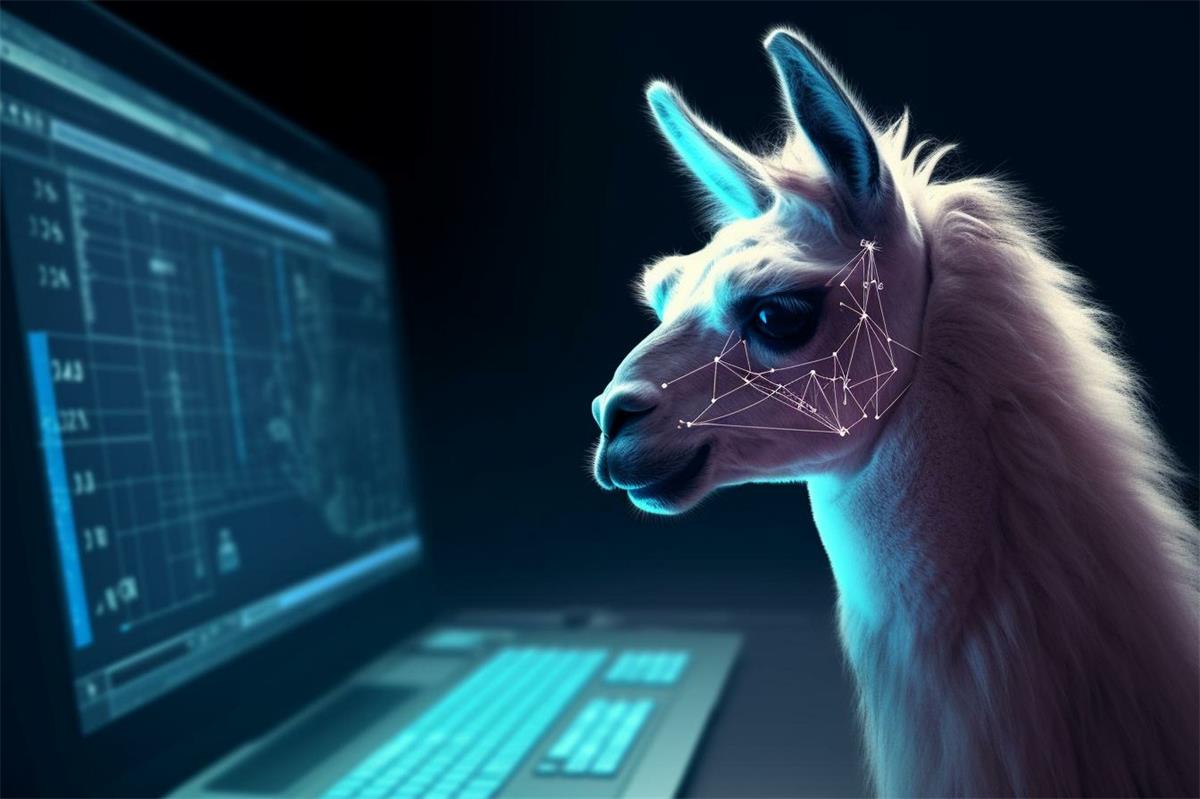
Time series data, characterised by continuous and varied nature, poses challenges for transformers. IBM Research has been a trailblazer in this field, introducing the Time Series Transformer (TST) at KDD 2021. This sparked a "battle of time series transformers," with subsequent models like PatchTST and PatchTSMixer outperforming traditional methods by up to 60%.
IBM's foray into adapting pre-trained Large Language Models (LLMs) for time series forecasting is another frontier. While LLMs are typically slow due to their size and single-variable focus, IBM's Tiny Time Mixers, a faster foundation model with nearly 1 million parameters, promises speed and flexibility.
The real-world applications are diverse, from optimising cement production to exploring the mysteries of the universe. IBM envisions a transformer capable of extracting dynamic event structures across domains, paving the way for accurate predictions in uncharted territories.
"Time series data has no common dictionary of patterns across domains," says IBM's Vijay Ekambaram. The challenge is immense, but the potential rewards are significant. As IBM continues to push the boundaries, the prospect of more accurate forecasts holds tremendous value for enterprises across industries.
#GenerativeAI