Advances in artificial intelligence, particularly in connection with the advent of applications using generative AI, have opened awareness of the security and ethical issues of this rapidly expanding field. Although the subject has been discussed for a long time by solution providers such as IBM and others, it is this leap forward that we have experienced over the last 3 years that has allowed a real awareness among governments and users about the risks inherent in the unbridled use of AI. In connection with the "black box" nature of the latest advances, as well as the fact that these technologies have been freely put in the hands of users without any particular knowledge in the field, in particular prior training in ethical issues.
In this "wild west" that is still artificial intelligence in many fields, there are many grey areas, and the rules are established as progress is made. Whether it is in terms of the inappropriate use of personal data, particularly socio-demographic data; the development of biased models; or through the use of uncontrolled technologies, which sometimes also have a high environmental cost; In short, the risks are numerous. While waiting for clear regulations to be put in place, organizations involved in AI must self-regulate and put in place processes and methods that ensure quality AI, in complete safety and in compliance with the clear ethical framework.
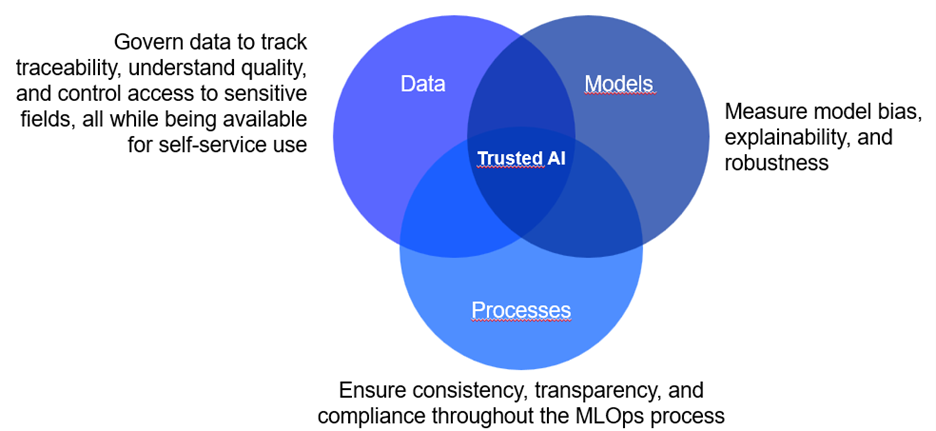
Such a practice of artificial intelligence is possible, and follows 3 major dimensions:
- Data governance: govern data to track lineage, understand data quality, and control access, including sensitive fields, masking it as needed, all while ultimately being available for self-service use across the organization.
- Model governance: list the models, their authors, and the associated data. But also, to ensure their transparency, explainability and absence of bias.
- Framework processes: ensure consistency, transparency, and compliance throughout the MLOps process. Enable risk and cybersecurity teams to take part in the AI effort by auditing models.
In practice, 5 pillars must be respected when implementing a trusted AI, pillars on which IBM has built its products and frameworks:
- Transparency: open to inspection.
- Explainable: Easy-to-understand results and decisions.
- Fair: Impartiality and mitigated bias.
- Robust: Effectively handles exceptional conditions and minimizes safety risks.
- Private: Powered by high-integrity, standards-compliant data.
The people involved, directly or indirectly, in the field of AI, and the related field of advanced analytics, are increasingly distant from historical teams. While AI and advanced analytics have long been confined to the development and deployment of models, therefore linked to data science teams, in particular the Chief-Data-Officer (CDO); we are seeing more and more the involvement of cybersecurity teams (linked to the CISO) and risk management teams (via the CRO). This change is major for practitioners, who must deliver quality projects, with a good return on investment, but also safe from a security point of view, and finally not exposing the company's data or assets.
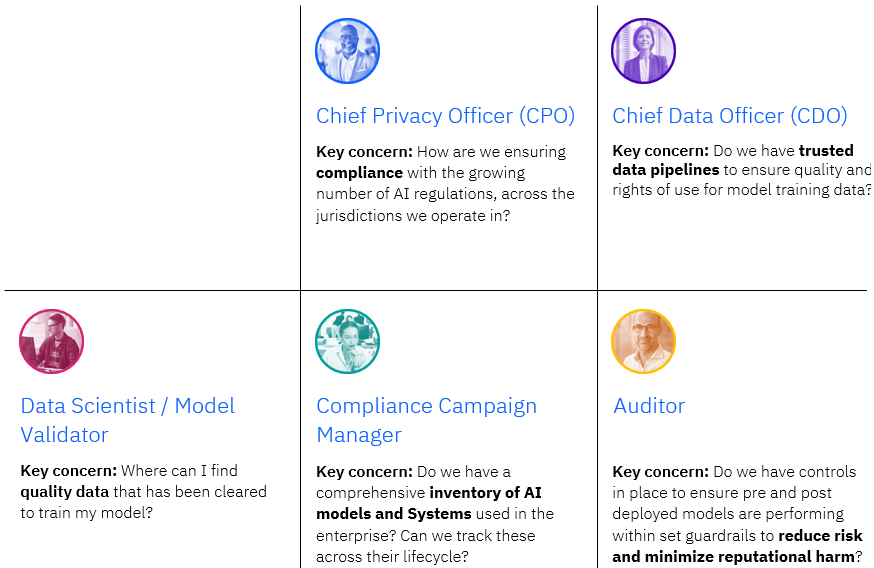
These people are asking themselves increasingly complex and stressful questions in some cases:
· Who uses open-source software? Which libraries? Are these libraries reliable?
· How many models are there in production?
· What data were used for these models?
· Is its data reliable? Masked? Unbiased?
· What is the risk exposure to the organization?
· Are my models still performing well in production?
· Which LLMs are used?
· Are our users exposing confidential data via RAG?
IBM's fundamental research (IBM Research) and product teams have been working on the subject for several years and developed a series of framework processes (open-source) as well as products (such as watsonx.gov), ready to be used by researchers, companies and governments, in order to ensure the creation of safe and ethical AI. These solutions automate end-to-end MLops and AI governance processes. Their routines provide a reliable view of the AI footprint in the organization. Reports are issued to decision-makers, providing information regarding the organization's AI exposure.
In conclusion, the AI wave will not stop, and we must all change our practices in order to support it, not restrict it, by keeping it within ethical and safe frameworks. Fortunately, technologies such as watsonx.governance exist to facilitate the mission of the various teams directly or indirectly linked to the management of AI in organizations, whether private or public.
See watsonx.governance in action: https://mediacenter.ibm.com/media/Meet+watsonx.governance/1_72hhrkxl
Key points :
- AI governance is imperative and not incidental. There is never a reliable AI, especially without having the various necessary frameworks in place such as data governance and model governance.
- The risks associated with biased or poorly designed AI are major, whether in monetary, ethical or image terms.
- Ethical AI has 5 major pillars: transparency, explainability, impartiality, robustness and privacy.
- The implementation of governance and ethics in AI is greatly simplified by the purchase of dedicated solutions.
Advice : Don't delay in addressing the issue of AI ethics in your organization. Whether it's through a simple internal alignment meeting, or through the purchase of dedicated solutions that automate everything for you. IBM offers free governance projects to help you start and get a foot in the door.