Originally posted by: sco1
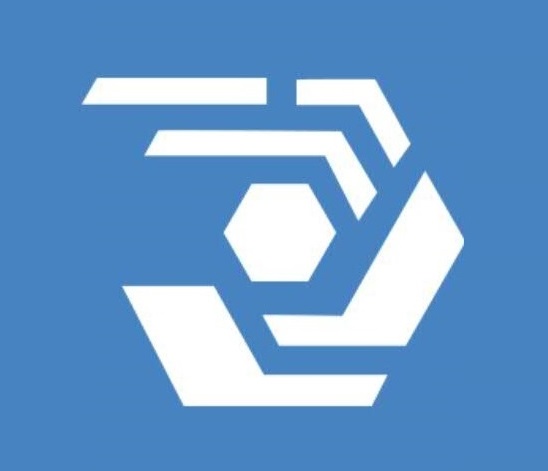
Author: Sum Huynh
Published on January 23, 2018 / Updated on January 25, 2018
IBM Spectrum Conductor Deep Learning Impact 1.1 provides many deep learning (DL) features, including: dataset management, hyperparameter configuration and tuning, training monitoring and optimization (DL Insight), native distributed training, and IBM Fabric distributed training. This blog shows how to use the IBM Spectrum Conductor Deep Learning Impact API in community TensorFlow models to take advantage of these features. It covers:
-
Downloading a community TensorFlow model
-
Renaming the main file in the TensorFlow model
-
Using a dataset with the TensorFlow model
-
Preparing the TensorFlow model for configurable hyperparameters
-
Preparing the TensorFlow model for hyperparameters tuning
-
Preparing the TensorFlow model for DL Insight
-
Preparing the TensorFlow model for IBM Fabric
As this blog focuses on the programming aspect of TensorFlow models, it is important that the reader is familiar with Python, TensorFlow, IBM Spectrum Conductor with Spark and IBM Spectrum Conductor Deep Learning Impact.
Prerequisites
- An IBM Conductor with Spark 2.2.1 cluster with IBM Spectrum Conductor Deep Learning Impact 1.1.0 add-on installed and configured correctly. For more information about installing and configuring IBM Spectrum Conductor Deep Learning Impact 1.1.0, see IBM Knowledge Center at http://www.ibm.com/support/knowledgecenter/SSWQ2D_1.1.0/in/installation.html. To try a free version of IBM Spectrum Conductor Deep Learning Impact, download the evaluation copy.
- At least one Spark instance group (SIG) created and started in the IBM Spectrum Conductor with Spark cluster.
Step 1: Downloading a community TensorFlow model
- Obtain a community TensorFlow model from. For the purposes of this blog, we will use Cifar10 as an example model. Download the Cifar10 model from the TensorFlow community, here: https://github.com/tensorflow/models/tree/master/tutorials/image/cifar10. We will need the following files:
- Cifar10.py
- Cifar10_input.py
- Cifar10_train.py
- Follow the steps here https://www.tensorflow.org/deploy/distributed to update Cifar10_train.py to run distributed (or search the community for a distributed version for example here: https://stackoverflow.com/questions/42707868/tensorflow-cifar10-distributed-training-accuracy). Once the distributed version has been run and tested successfully by itself (outside IBM Spectrum Conductor Deep Learning Impact), follow the following steps to enhance it to take advantage of the IBM Spectrum Conductor Deep Learning Impact features.
Step 2: Renaming the main file in the TensorFlow model
As seen on the model creation page in the cluster management console, IBM Spectrum Conductor Deep Learning Impact supports some distributed training engine options.
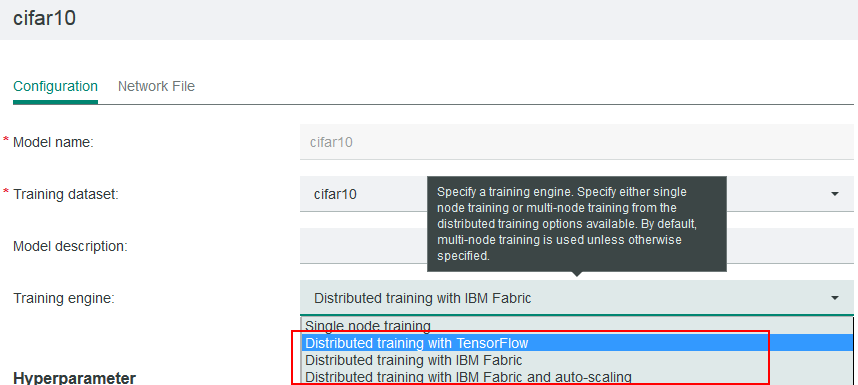
For the native distributed training option, Distributed training with TensorFlow, IBM Spectrum Conductor Deep Learning Impact expects a main.py file which it calls for training. For Cifar10, you must rename the Cifar10_train.py file to main.py.
For the IBM Fabric distributed training options, Distributed training with IBM Fabric and Distributed training with IBM Fabric and auto-scaling, IBM Spectrum Conductor Deep Learning Impact expects a fabricmodel.py file which it calls for training. Make sure to create a new file called fabricmodel.py in the same location as the main.py file. More information about the fabricmodel.py file can be found in Step 7.
For Cifar10, your directory should look like this:

Step 3: Using a dataset with the TensorFlow model
IBM Spectrum Conductor Deep Learning Impact supports different DL data formats (LMDB, TFRecord), can ingest raw images, and provides dataset management. This step shows how to use DL datasets in a TensorFlow model. Here, we assume that you already have an appropriate dataset created. For example, you might have a cifar10 dataset, as follows:
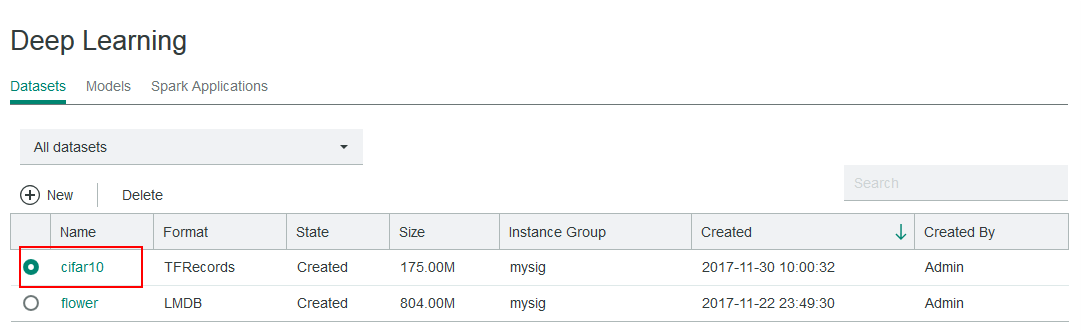
The dataset (cifar10 in this case) must also be used by the model that you created. Make sure that the model you created points to the directory containing your model, you can set your model to point to the dataset as follows:
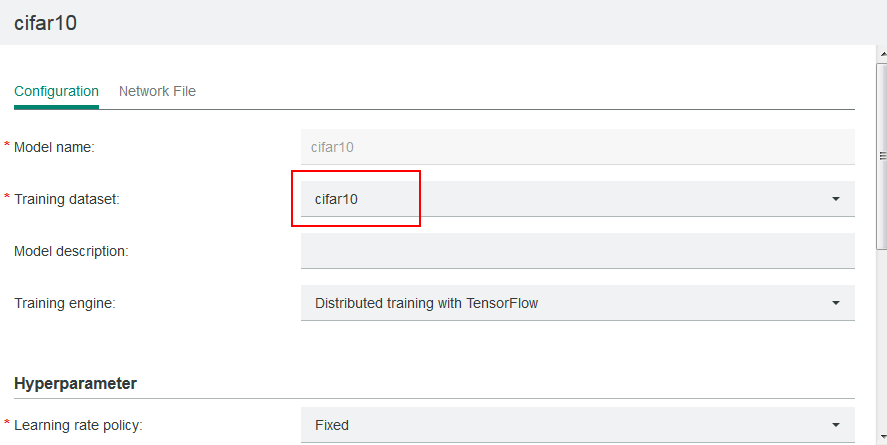
Next, modify the model to make it call the IBM Spectrum Conductor Deep Learning Impact API to obtain the dataset. IBM Spectrum Conductor Deep Learning Impact provides a Python module called tf_parameter_mgr which you can use to refer to IBM Spectrum Conductor Deep Learning Impact datasets. For the Cifar10 example, all changes to refer to the dataset will be in the cifar10_input.py file. Here is how to use it:
- Import the module:
Import tf_parameter_mgr
- tf_parameter_mgr.getTrainData() returns the names of all the dataset files used for training. You will need to call this function and pass the results to where the model is reading input from. For Cifar10, the changes in the cifar10_input.py file are as follows (old code is commented out):
…
# filenames = [os.path.join(data_dir, ‘data_batch_%d.bin’ %i) for I in xrange(1,6)]
filenames = tf_parameter_mgr.getTrainData()
filename_queue = tf.train.string_input_producer(filenames)
…
- The functionality of tf_parameter_mgr.getTestData() is similar to getTrainData(), however, tf_parameter_mgr.getTestData() returns the names of all the dataset files used for testing. For Cifar10, the changes are as follows:
…
if not eval_data:
# filenames = [os.path.join(data_dir, ‘data_batch_%d.bin’ %i) for I in xrange(1,6)]
filenames = tf_parameter_mgr.getTrainData()
…
else:
# filenames = [os.path.join(data_dir, ‘test_batch.bin’)]
filenames = tf_parameter_mgr.getTestData()
…
With the changes so far, you should be able to train the model with the “Distributed training with TensorFlow” training engine, however, since all the hyperparameters are still hardcoded in the model you cannot do hyperparameter tuning or use DL Insight.
Step 4: Preparing the TensorFlow model for configurable hyperparameters
Instead of hardcoding hyperparameters in models, you can configure hyperparameters from the IBM Spectrum Conductor Deep Learning Impact cluster management console. Assume that you set the hyperparameters for your model as shown below. This section shows how to modify the model to call the IBM Spectrum Conductor Deep Learning Impact API to pick up these hyperparameters:
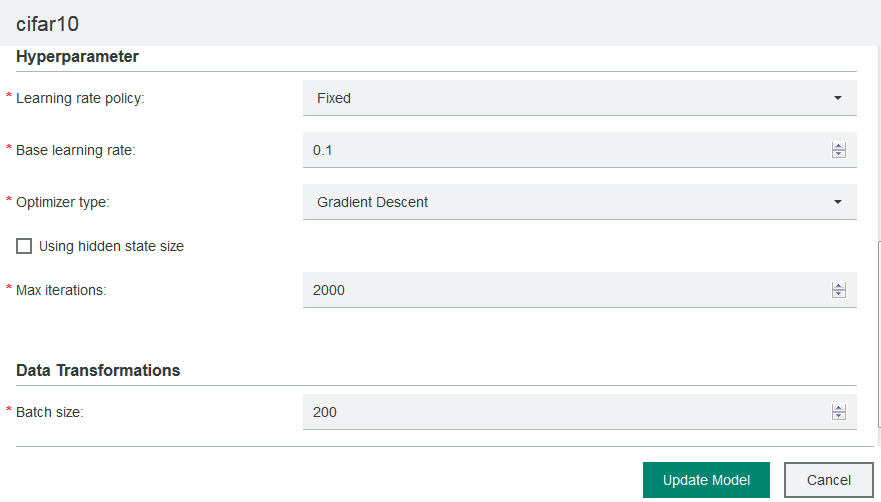
The tf_parameter_mgr module provides the function to get hyperparameters. For Cifar10, the changes below need to be made to the main.py file. Here is how to use it (original code commented out):
Import tf_parameter_mgr
#tf.app.flags.DEFINE_integer('max_steps', 1000, """Number of batches to run.""")
FLAGS.max_steps = tf_paramter_mgr.getMaxSteps()
#INITIAL_LEARNING_RATE = 0.1 # Initial learning rate.
INITIAL_LEARNING_RATE = tf_parameter_mgr.getBaseLearningRate()
#LEARNING_RATE_DECAY_FACTOR = 0.1 # Learning rate decay factor.
LEARNING_RATE_DECAY_FACTOR= tf_parameter_mgr.getLearningRateDecay()
FLAGS.batch_size= tf_paramter_mgr.getTrainBatchSize()
# opt = tf.train.GradientDescentOptimizer(lr)
opt = tf_parameter_mgr.getOptimizer(lr)
Step 5 : Preparing the TensorFlow model for hyperparameters tuning
Once the hyperparameters are configurable, IBM Spectrum Conductor Deep Learning Impact can automatically tune these hyperparameters to find the best ones and generate a model with those best hyperparameters. This step shows how to prepare the model to log information so that IBM Spectrum Conductor Deep Learning Impact can use it to perform hyperparameter tuning as shown in the cluster management console here:
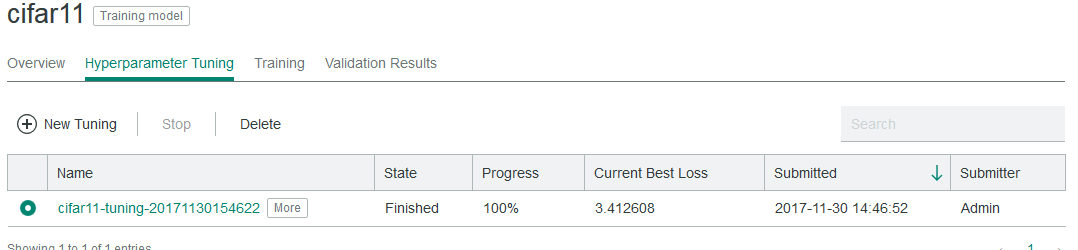
Note that you only have to do this step for main.py (for training engine ‘Distributed training with TensorFlow’), not for fabricmodel.py (for training engine ‘Distributed training with IBM Fabric’ or ‘Distributed training with IBM Fabric auto-scaling’) since IBM Fabric already does this automatically.
IBM Spectrum Conductor Deep Learning Impact provides a Python module called monitor_cb. For the Cifar10 example, all changes referring to dataset are in the main.py file. Here is how to use it:
import monitor_cb
…
# Calculate loss.
loss = cifar10.loss(logits, labels)
…
If is_chief:
log_dir = os.path.join(FLAGS.train_dir, ‘log’)
monitor = monitor_cb.CMonitor(log_dir, tf_parameter_mgr.getTestInterval(), tf_parameter_mgr.getMaxSteps())
…
monitor.SummaryScalar(“train loss”, loss)
monitor.SummaryScalar(“test loss”, loss)
train_summaries = tf.summary_merge_all(monitor_cb.DLMAO_TRAIN_SUMMARIES)
test_summaries = tf.summary_merge_all(montor_cb.DLMAO_TEST_SUMMARIES)
Step 6: Preparing the TensorFlow model for DL Insight
With IBM Spectrum Conductor Deep Learning Impact, you can monitor the training progress of your model. If training is not going well due to an exception, divergence, under-fitting or over-fitting, then the error is flagged and a suggestion for how to correct it will be available in the DL Insight page of the cluster management console. As an example, here are some charts from DL Insight:
This step shows how to update the model to enable DL Insight and have the insight metrics show up in the cluster management console. The changes are in addition to the changes for hyperparameter tuning, and repeat here for clarity.
For Cifar10, the code below shows how to get a weight historgram for each layer as shown in a screenshot above:
import monitor_cb
…
If is_chief:
log_dir = os.path.join(FLAGS.train_dir, ‘log’)
monitor = monitor_cb.CMonitor(log_dir, tf_parameter_mgr.getTestInterval(), tf_parameter_mgr.getMaxSteps())
…
loss= …
accuracy = …
train_op = …
if is_chief:
graph = tf.get_default_graph()
for layer in [‘conv1’, ‘conv2’, ‘local3’, ‘local4’]:
monitor.SummaryHist(‘weight’, graph.get_tensor_by_name(layer+ ‘/weights:0’), layer)
monitor.SummaryHist(‘bias’, graph.get_tensor_by_name(layer+ ‘/biases:0’), layer)
monitor.SummaryHist(‘activation’, graph.get_tensor_by_name(layer + ‘/’ + layer + ‘:0’), layer)
monitor.SummaryNorm2(“weight”, graph.get_tensor_by_name(layer+ ‘/weights:0’), layer)
monitor.SummaryGradient(“weight”, loss)
monitor.SummaryGWRatio()
monitor.SummaryScalar(“train loss”, loss)
monitor.SummaryScalar(“train accuracy”, accuracy)
monitor.SummaryScalar(“test loss”, loss)
monitor.SummaryScalar(“test accuracy”, accuracy)
train_summaries = tf.summary_merge_all(monitor_cb.DLMAO_TRAIN_SUMMARIES)
test_summaries = tf.summary_merge_all(montor_cb.DLMAO_TEST_SUMMARIES)
Step 7: Preparing the TensorFlow model for IBM Fabric
IBM Spectrum Conductor Deep Learning Impact provides the ability to auto-scale distributed training. In addition to being able to scale up and down as resources become available, this type of training is more robust as it can handle worker crashes. When you select IBM Fabric or IBM Fabric with auto scaling training engine as shown below, IBM Spectrum Conductor Deep Learning Impact runs the fabricmodel.py file.
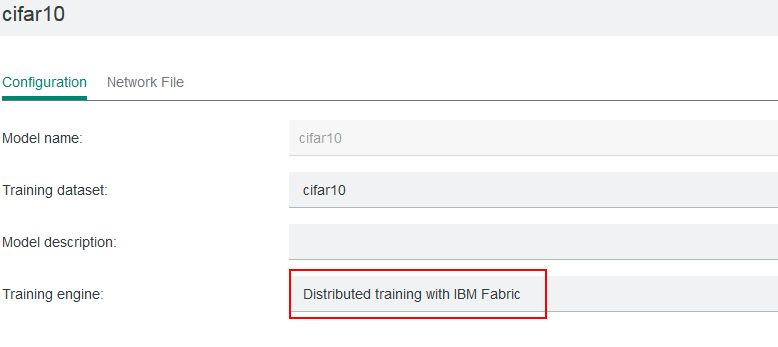
You can use the meta_writer module provided by IBM Spectrum Conductor Deep Learning Impact to implement fabricmodel.py. The main part of fabricmodel.py is:
from meta_writer import *
…
Def main(argv=None):
…
Write_meta(tf, None, accuracy, loss, eacuracy, eloss, optApplyOp, grads_and_vars, global_step, FLAGS.model_file, FLAGS.meta_file, FLAGS.graph_file, restore_file, checkpoint_file, snapshot_interval)
if __name__ == ‘__main__’:
tf.app.run()
- FLAGS.model_file, FLAGS.meta_file, FLAGS.graph_file, restore_file (or FLAGS.weights) are dynamically generated and passed in by IBM Spectrum Conductor Deep Learning Impact.
- checkpoint_file = os.path.join(FLAGS.train_dir, “model.ckpt”)
- snapshot_interval: for Cifar10, this is set to 100.
Once the main part of fabricmodel.py has been updated, the rest of the parameters must also be updated similar as below – with original code commented out:
images, labels = cifar10.distorted_inputs()
eimages, elabels = cifar10.inputs(True)
global_step = tf.contrib.framework.get_or_create_global_step()
logits = cifar10.inference(images)
loss = cifar10.loss(logits, labels)
top_k_op = tf.nn.in_top_k(logits, labels, 1)
# accuracy = tf.reduce_mean(tf.cast(np.sum(top_k_op), tf.float32)
accuracy = tf.reduce_mean(tf.cast(top_k_op, "float"), name="accuracy")
# train_op = cifar10.train(loss, global_step)
optApplyOp, grads_and_vars = cifar10.train(loss, global_step, True)
tf.get_variable_scope().reuse_variables()
elogits=cifar10.inference(eimages)
eloss = cifar10.loss(elogits, elabels)
etop_k_op = tf.nn.in_top_k(elogits, elabels, 1)
eaccuracy = tf.reduce_mean(tf.cast(etop_k_op, "float"), name="eaccuracy")
checkpoint_file = os.path.join(FLAGS.train_dir, "model.ckpt")
restore_file = FLAGS.weights
snapshot_interval = 100
write_meta(tf,None,accuracy,loss, eaccuracy, eloss, optApplyOp, grads_and_vars,global_step,FLAGS.model_file, FLAGS.meta_file,FLAGS.graph_file,restore_file,checkpoint_file,snapshot_interval)
Summary
In summary, this blog shows step by step how to modify a TensorFlow model to take advantage of the deep learning features provided by IBM Spectrum Conductor Deep Learning Impact.
To see more examples of models that are already modified for IBM Spectrum Conductor Deep Learning Impact and ready to be used, see our ibmcws-deep-learning-caffe-samples repository for Caffe samples and ibmcws-deep-learning-tensorflow-samples for TensorFlow samples.
For more information about IBM Spectrum Conductor Deep Learning Impact, see:
www.ibm.biz/DeepLearningImpact
#SpectrumComputingGroup